Zach Grey, a researcher at NIST, is designing better wind turbines using sophisticated math.
R. Jacobson/NIST is credited.
A wind turbine gently turning in the breeze has a wonderful simplicity to it.  ,
A rotating force is produced as the wind passes by the turbine’s blades, spinning the enormous assembly. The rotation is then transformed, just like traditional power generation, into electricity.  ,
A wind turbine is made up of three blades that are bent and twisted into teardrop-like shapes. On one edge, the turbine blades are cylindrical, and on the other, they are sharper.  ,

The wind turbine shapes change as they move from the hub of the turbine blade ( left ) to the tip on the right in this blade graphic.
Credit: NIST
Over its long, thin axis, the blade’s surface changes. It starts out with thick, blunt shapes and ends up with thin, slender teardrop shapes. ( For a more detailed view of the shifting pattern of shapes moving from the hub on the left side to the tip in the right side, see the blade graphic above. ) Therefore, a turbine spinning in the wind may appear straightforward, but designing and measuring wind turbine blades, using an array of shifting cross-sectional shapes, calls for extensive use of sophisticated geometry.  ,
I work with a group that studies geometric method abstractions to create better wind turbines using mathematics. We intend to use our techniques in the future to evaluate the efficiency of wind turbine production and field performance.  ,
Since design and measurement are intertwined, tackling both of their challenges will help us move toward a future with cleaner energy.  ,
We can create computer models to forecast how power is produced by air flowing around a spinning turbine’s blades using the laws of physics. The forces that rotate the turbine change along with the blade’s surface.  ,
Aerodynamics is the study of how these forces change when the surface changes. Similar to how an airplane’s wings must be designed to keep us aloft during flight, the aerodynamic shape of a turbine blade is essential to maximizing the power out of each turbine.
Lift and drag are two of the most significant forces at work on aerodynamic surfaces. We refer to it as lift because the force defying gravity with an aircraft wing is the same force that spins a turbine. Drag is the force that slows the rotation of an aircraft or turbine that is perpendicular to lift.  ,
We can research how to best design the cross-sectional shapes of turbine blades known as airfoils using physics-based models. The graphic includes a few illustrations of wind turbine airfoils. Airfoils are the solid, black outlines of cross-sections. They resemble bread slices that have been cut from a massive loaf in the shape of bluff. More lift is produced with less drag by better airfoil shapes.  ,
The airfoil shapes of a turbine blade are fixed, whereas an aircraft wing has control surfaces that the pilot can alter. For wind turbines, the inner portion supports the spinning blade while the outer portion produces the most lift. Therefore, more power is produced by improved airfoil shapes with greater lift near the tip and less drag close to the hub.  ,
The final blade surface, which is determined by a series of airfoil shapes, must then be produced, activated, and measured while in use. How we measure the shape in practice has significant effects on how we represent it for design. And NIST focuses on overcoming obstacles using measurement science.
How do we measure and mathematically define shape is the key question for NIST. Can the best wind turbine be designed and measured using our work?
Geometry Causes the Turbine to Rotate
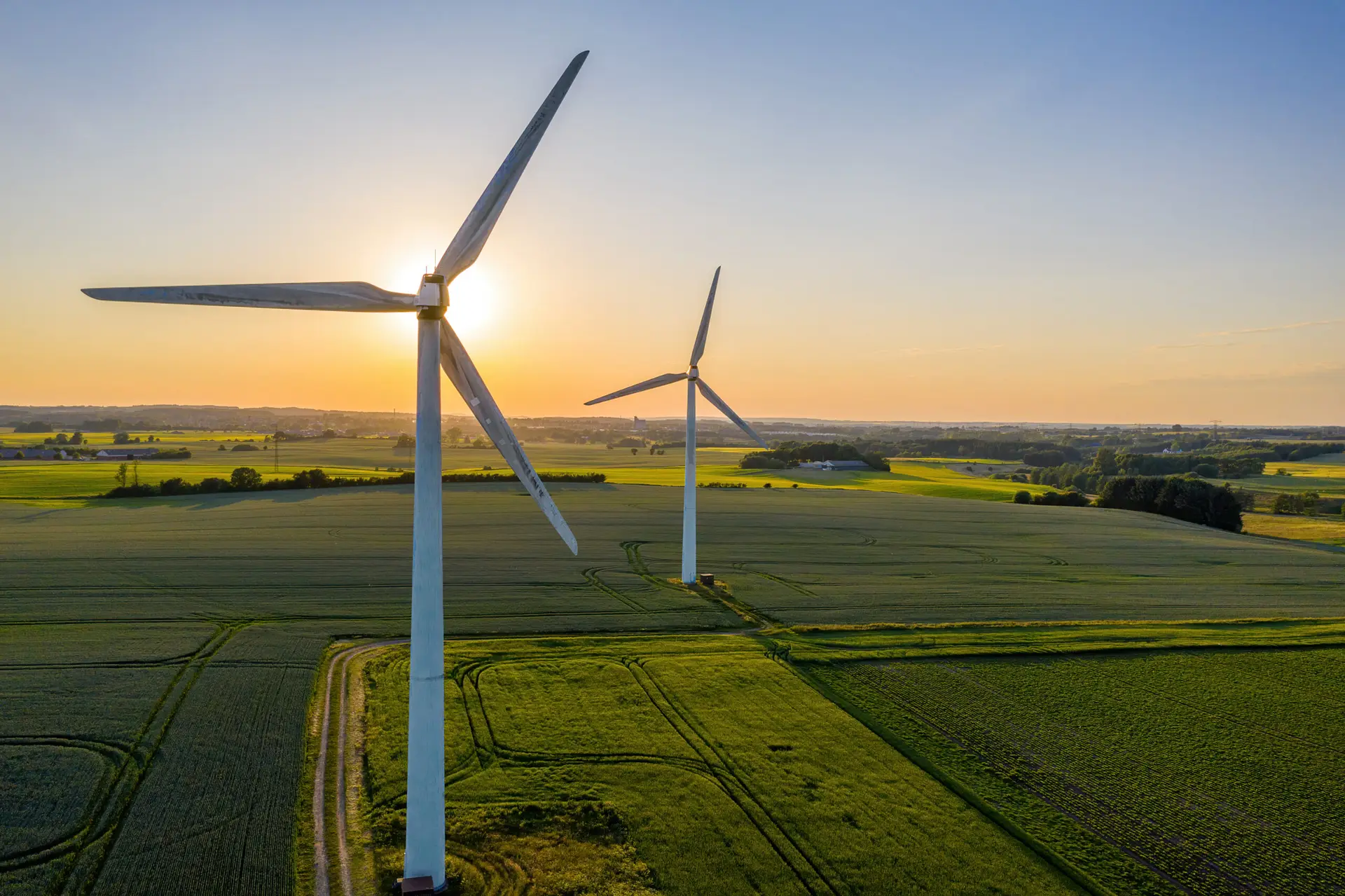
Physics and math are necessary for designing wind turbines. The laws of physics are used by computer models to forecast how power will be produced by air flowing around a spinning turbine’s blades.
Credit: Shutterstock/Nick Brundle Photography
We are creating new techniques to describe and generate these complex airfoil shapes in collaboration with the National Renewable Energy Laboratory ( NREL ) in order to develop next-generation designs and improve measurements in the field.  ,
As a first step, we’ve addressed the issue with turbine blade design. To meet structural requirements, we aim to produce power that is as efficient as possible while remaining within size constraints. I’ll use a few examples from the scientific method to explain this.  ,
According to the theory, separating each airfoil’s rotation and size from all other shape-related changes can result in better designs and measurements with fewer overall numbers—what we refer to as parameters—that change how the shape is represented in a computer. We change the parameters in order to alter the shapes. The math problem gets trickier the more you have. Traditional methods require between 100 and 200 parameters in total to define a blade!  ,
It’s a straightforward idea. We mathematically “divide these effects out” and study what is left over as learned from data if we want precise control over particular changes, such as rotation and the size of each airfoil. Fewer requirements make it much simpler for us to comprehend modifications to the design.  ,
With the help of airfoil shapes gleaned from NREL shape data, our applied geometric approach offers a fresh approach to defining and examining this concept.
The experiment: The design strategy used at NREL involves resolving an inverse problem, one of the most challenging math problems. We need “XYZ” aerodynamics, according to an inverse problem, which determines the desired outcomes. In order to find a group of airfoils that meet the required aerodynamics, we then proceed backward through the modeled physics. NIST mathematicians frequently consider inverse problems for measurement, and they can be very difficult to solve if we are able to do so at all!
First, we use NREL shape data to train our geometry model, which results in tens of thousands of new shapes. Then, we forecast the aerodynamics of each shape using physics-based models. Finally, our NREL colleagues train the AI to instruct the geometry model on how to use the generated data to generate new designs. This effectively” closes the loop” in the analysis.
The outcomes: NREL was able to create new wind turbine designs to lower the cost of energy by successfully utilizing the geometric techniques in a tool called G2Aero. More on this later. Because we carefully crafted the descriptions of the shapes, we also have faith in the AI’s findings. As a result, AI only offers suggestions for new shapes that are represented in our way. Most importantly, our geometry approach stops AI from producing irrational, wiggling shapes that are n’t suitable for modeling physics or aerodynamics.  ,
Additionally, the conventional method, which involved hundreds of parameters, was shortened to just four parameters to describe an entire blade! This is entirely attributable to our data-driven geometry model, notAI. We can train the AI more easily with such a significant decrease in the overall parameter count. Additionally, we can compare different methods for mapping requirements to parameters and better checks and balances ( constraints ) on AI recommendations.
Future applications of this research are numerous and exciting. For instance, if wind turbines are offshore, they are frequently monitored remotely and only serviced when a problem is found. Using our geometry and physics-based modeling, we hope to improve remotely measuring turbines in use to ensure that they are operating effectively.
We could use physics to look back in time to see what has changed in the environment if we notice a change in power generation. For instance, the blade could be harmed or there might be an accumulation of dirt, salt, or sediment that would affect the aerodynamics of the turbine blade.  ,
The design and measurement of the large spinning blades are just the beginning of this geometry research. From designing floating offshore platforms for future wind turbines to measuring microscopic shapes hidden in the materials used to manufacture blades, we think we can improve almost every aspect of wind-generated power for less money.
There are issues everywhere that call for precise definition and measurement of shapes. Beyond designing and measuring wind turbines, geometry-based research has a wide range of applications.
Making Things Better With Math
During my undergraduate studies at Embry- Riddle Aeronautical University, I developed a love for applied math. I began designing jet engines at the Rolls- Royce Corporation after receiving my degree, not the cars.  ,
Teaching designers and analysts how to incorporate statistical factors into their intricate physics-based modeling was one of my jobs. My love of using applied math to develop new perspectives on issues and strengthen things was further fueled by these experiences.
The fact that universal principles remain the same while the technology and applications of those principles are constantly evolving is what I find most fascinating about studying applied math. For me, mathematics is the art of giving language to logic in many ways. Math provides explanations and interpretations for a wide range of natural phenomena. The mathematics almost certainly contain the solution if you’re looking for an explanation or interpretation of any physical or artificial process.
One of the most enjoyable aspects of my work is using general math principles in various situations. For instance, the same geometry techniques we applied to wind turbines can also be used to explain the intricate microstructures present in lithium-ion and steel batteries. More ideal telecommunications processes can be described from other geometric angles.  ,
I am able to use these abstract, foreign concepts in this kind of research to address issues and questions in the real world, such as:
-  ,” How do we construct the best wind turbine?”
- ” Why did that AI model choose that course of action?”
- What distinguishes one material’s measurement from another statistically? 
In order to better understand the complex nature of shape in data, this geometry-focused research provides us with a wealth of explanations and interpretations of numerous different problems.
Future Planning for a Clean Energy Future
I’m excited to contribute to how we can produce clean energy in the future in addition to studying difficult math problems.  ,
( 128 million people, excluding Alaska ), make up about 40 % of the country’s population who reside in coastal counties. How we produce the energy, as well as how we deliver it, is one of the biggest challenges facing future energy generation.  ,
The country’s center, where the wind blows the most on land, has an increasing number of wind turbines, but it is difficult to deliver that wind power to the coastal regions due to infrastructure issues. Additionally, offshore turbines can be much larger, with nearly three times the potential power generation of those based on land. Additionally, it is anticipated that the wind will blow faster and more frequently offshore near coastal areas.  ,
These factors obviously increase the need for offshore wind power, but they also lead to more difficult design and measurement issues. For future generations of offshore applications—a significantly more volatile environment with a lot of severe weather—we want to develop the most effective wind turbine systems and make them strong and resilient.  ,
assisting with the design of next-generation turbines is only the first step. In order to ensure that the offshore turbines are resilient to the test of time and can still supply power to those who require it, we must also measure them while they are in use.
According to NREL, new designs could achieve about a 1 % reduction in cost of energy if we could redesign existing wind turbines using our geometry model. Even though it may not seem like much, if we could redesign every turbine already in use, we would be able to save about$ 288 million in 2021 alone.
However, by 2050, wind energy production is anticipated to quadruple. In a very conservative estimate, implementing these ostensibly modest 1 % savings over the course of the following 30 years could result in overall savings of billions of dollars when compared to current techniques.
If we assume that offshore wind turbines only produce electricity 50 % of the time, we anticipate that our new methods can save Americans$ 60 million per year in energy costs based on current energy goals. The overall savings with our next-generation wind turbine designs could be between$ 120 and$ 180 million per year, which is a very conservative estimate.  ,
The improvement of energy costs will be significantly impacted by addressing the design and measurement issues for wind turbine blades. Along with NREL scientists, NIST mathematicians are prepared to address these problems in the direction of the promise of clean, efficient energy.  ,
It feels fantastic to contribute to creating a future using clean, dependable energy sources. With a changing global economy and climate, we face many difficulties.  ,
I’m proud of the contributions we’ve made to the wealth of scientific discoveries aimed at a safer, more stable, and cleaner future for the country, even though wind energy is only one component of an extremely complex puzzle.
Special thanks to Andrew Glaws and Ganesh Vijayakumar at NREL for providing estimates summarizing the outcomes of using our geometry methods, along with the other members of the ARPA- E DIFFERENTIATE team, including Ryan King, Olga Doronina, James Baeder, and Bumseok Lee.